ptrade策略代码:集合竞价追涨停策略
这个是示例代码,我们来大体讲解一下:
分解讲解:
initialize是初始化函数,一定要有的函数。在策略运行时首先运行的,而且只会运行一次。
set_universe(g.security) 在把标的代码放进去。这里是 '600570.SS' 记得要有后缀,上证股票用 .SS ,深圳股票用.SZ。
run_daily(context, aggregate_auction_func, time='9:23')
这一行是设定每天运行一次。这个策略是日线级别的,所以每天只要运行一次就可以了。 分别传入3个参数。
第一个参数固定是context,第二个要执行的函数名,记住只能传函数名,不能把括号也加进去,第三个参数,是运行的时间,现在设定在 9:23
那么接下来就是要实现上面那个函数名了: aggregate_auction_func,这个名字可以随意定义
stock = g.security , g是全局变量,用来在上下文中传递数据。这里就是上面的'600570.SS'
snapshot = get_snapshot(stock)
这个就是获取行情数据,当前的价格
up_limit = snapshot[stock]['up_px']
拿到这个标的的涨停价
if float(price) >= float(up_limit):
这个是当前价格大于涨停板价格(主要考虑了四舍五入,用于大号比较安全)
order(g.security, 100, limit_price=up_limit)
这个就是下单函数。
买入100股,不限价,市价成交。
然后就可以点击运行交易。
程序每天都会自动交易。

欢迎关注公众号
收起阅读 »
def initialize(context):
# 初始化此策略
# 设置我们要操作的股票池, 这里我们只操作一支股票
g.security = '600570.SS'
set_universe(g.security)
#每天9:23分运行集合竞价处理函数
run_daily(context, aggregate_auction_func, time='9:23')
def aggregate_auction_func(context):
stock = g.security
#最新价
snapshot = get_snapshot(stock)
price = snapshot[stock]['last_px']
#涨停价
up_limit = snapshot[stock]['up_px']
#如果最新价不小于涨停价,买入
if float(price) >= float(up_limit):
order(g.security, 100, limit_price=up_limit)
def handle_data(context, data):
pass
分解讲解:
def initialize(context):
# 初始化此策略
# 设置我们要操作的股票池, 这里我们只操作一支股票
g.security = '600570.SS'
set_universe(g.security)
#每天9:23分运行集合竞价处理函数
run_daily(context, aggregate_auction_func, time='9:23')
initialize是初始化函数,一定要有的函数。在策略运行时首先运行的,而且只会运行一次。
set_universe(g.security) 在把标的代码放进去。这里是 '600570.SS' 记得要有后缀,上证股票用 .SS ,深圳股票用.SZ。
run_daily(context, aggregate_auction_func, time='9:23')
这一行是设定每天运行一次。这个策略是日线级别的,所以每天只要运行一次就可以了。 分别传入3个参数。
第一个参数固定是context,第二个要执行的函数名,记住只能传函数名,不能把括号也加进去,第三个参数,是运行的时间,现在设定在 9:23
那么接下来就是要实现上面那个函数名了: aggregate_auction_func,这个名字可以随意定义
def aggregate_auction_func(context):
stock = g.security
#最新价
snapshot = get_snapshot(stock)
price = snapshot[stock]['last_px']
#涨停价
up_limit = snapshot[stock]['up_px']
#如果最新价不小于涨停价,买入
if float(price) >= float(up_limit):
order(g.security, 100, limit_price=up_limit)
stock = g.security , g是全局变量,用来在上下文中传递数据。这里就是上面的'600570.SS'
snapshot = get_snapshot(stock)
这个就是获取行情数据,当前的价格
up_limit = snapshot[stock]['up_px']
拿到这个标的的涨停价
if float(price) >= float(up_limit):
这个是当前价格大于涨停板价格(主要考虑了四舍五入,用于大号比较安全)
order(g.security, 100, limit_price=up_limit)
这个就是下单函数。
买入100股,不限价,市价成交。
然后就可以点击运行交易。
程序每天都会自动交易。

欢迎关注公众号
收起阅读 »
ptrade如何以指定价格下单?
在正常handle_data 或者 run_interval 模式下下单,是无法指定价格的。
order函数:
order_target - 函数
order_value 函数
order_target_value - 函数
order_market 函数
上面几个在handle_data中使用的下单函数,都是无法指定价格的,limit_price 只是用于限价,比如你要卖1000股,limit_price的作用是不要把价格卖出你的目标,至于多少卖,是无法控制的。
但是有一个tick_data函数,专门用于行情交易的,里面可调用的函数也很少。
order_tick - tick行情触发买卖
注意到里面:
limit_price:买卖限价,当输入参数中也包含priceGear时,下单价格以limit_price为主
发现这里面居然可以定义价格下单,所以如果一定要指定价格,就需要使用tick_data 触发。
使用代码:
order函数:
order-按数量买卖
order(security, amount, limit_price=None)
买卖标的。
注意:
由于下述原因,回测中实际买入或者卖出的股票数量有时候可能与委托设置的不一样,针对上述内容调整,系统会在日志中增加警告信息:
根据委托买入数量与价格经计算后的资金数量,大于当前可用资金;
委托卖出数量大于当前可用持仓数量;
每次交易数量只能是100的整数倍,但是卖出所有股票时不受此限制;
股票停牌、股票未上市或者退市、股票不存在;
回测中每天结束时会取消所有未完成交易;
order_target - 函数
order_target(security, amount, limit_price=None)
买卖股票,直到股票最终数量达到指定的amount。
注意:该函数在委托股票时取整100股,委托可转债时取整100张。
参数
security: 股票代码(str);
amount: 期望的最终数量(int);
limit_price:买卖限价(float);
返回
Order对象中的id或者None。如果创建订单成功,则返回Order对象的id,失败则返回None(str)。
order_value 函数
order_value - 指定目标价值买卖
order_value(security, value, limit_price=None)
买卖指定价值为value的股票。
注意:该函数在委托股票时取整100股,委托可转债时取整100张。
order_target_value - 函数
order_target_value - 指定持仓市值买卖
order_target_value(security, value, limit_price=None)
调整股票仓位到value价值
注意:该函数在委托股票时取整100股,委托可转债时取整100张。
order_market 函数
order_market - 按市价进行委托
order_market(security, amount, market_type=None, limit_price=None)
可以使用多种市价类型进行委托。
注意:该函数仅在股票交易模块可用。
上面几个在handle_data中使用的下单函数,都是无法指定价格的,limit_price 只是用于限价,比如你要卖1000股,limit_price的作用是不要把价格卖出你的目标,至于多少卖,是无法控制的。
但是有一个tick_data函数,专门用于行情交易的,里面可调用的函数也很少。
tick_data(可选)里面下单,用的下单函数是
tick_data(context, data)
该函数会每隔3秒执行一次。
注意 :
该函数仅在交易模块可用。
该函数在9:30之后才能执行。
该函数中只能使用order_tick进行对应的下单操作。
order_tick - tick行情触发买卖
order_tick(sid, amount, priceGear='1', limit_price=None)
买卖股票下单,可设定价格档位进行委托
注意:该函数仅在交易模块可用。
参数
sid:股票代码(str);
amount:交易数量,正数表示买入,负数表示卖出(int)
priceGear:盘口档位,level1:1~5买档/-1~-5卖档,level2:1~10买档/-1~-10卖档(str)
limit_price:买卖限价,当输入参数中也包含priceGear时,下单价格以limit_price为主(float);
注意到里面:
limit_price:买卖限价,当输入参数中也包含priceGear时,下单价格以limit_price为主
发现这里面居然可以定义价格下单,所以如果一定要指定价格,就需要使用tick_data 触发。
使用代码:
def initialize(context):收起阅读 »
g.security = "600570.SS"
set_universe(g.security)
def tick_data(context,data):
security = g.security
current_price = eval(data[security]['tick']['bid_grp'][0])[1][0]
if current_price > 56 and current_price < 57:
# 以买一档下单
order_tick(g.security, -100, "1")
# 以卖二档下单
order_tick(g.security, 100, "-2")
# 以指定价格下单
order_tick(g.security, 100, limit_price=56.5)
def handle_data(context, data):
pass
国盛证券qmt mini模式 xtquant
国盛证券QMT:
【国盛QMT支持 xtquant qmt mini模式】
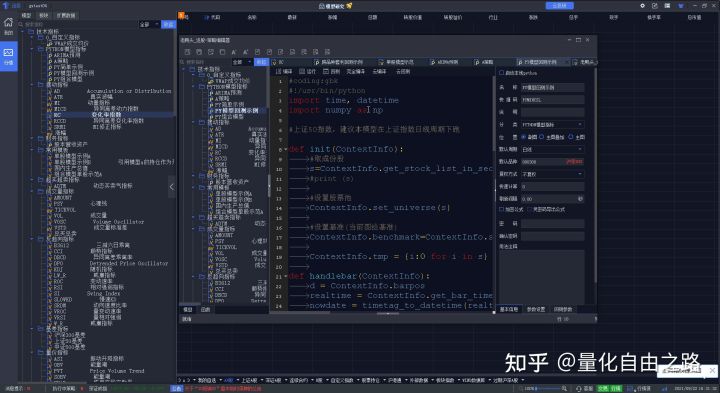
mini模式可以在外部运行,同时可以下载历史tick数据。
可以直接获取level2的数据
使用python代码直接运行,不用在qmt软件里面憋屈地写代码,可直接使用pycharm,vscode编写,且有代码提示,补全,好用多了。
附一个完整的策略例子。
保存为: demo.py
命令行下运行:
python demo.py
开通xtquant的方式可以咨询。
目前开户费率低,门槛低,提供技术支持与交流。
需要的朋友,可以扫码咨询:

备注开户 收起阅读 »
【国盛QMT支持 xtquant qmt mini模式】
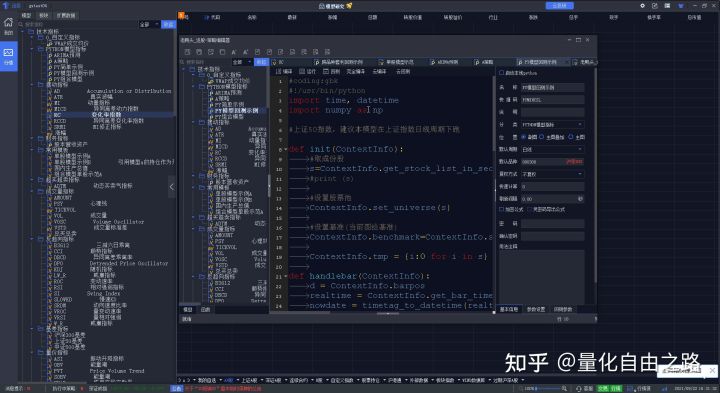
mini模式可以在外部运行,同时可以下载历史tick数据。
xtdata是xtquant库中提供行情相关数据的模块,本模块旨在提供精简直接的数据满足量化交易者的数
据需求,作为python库的形式可以被灵活添加到各种策略脚本中。
主要提供行情数据(历史和实时的K线和分笔)、财务数据、合约基础信息、板块和行业分类信息等通
用的行情数据
可以直接获取level2的数据
使用python代码直接运行,不用在qmt软件里面憋屈地写代码,可直接使用pycharm,vscode编写,且有代码提示,补全,好用多了。
附一个完整的策略例子。
保存为: demo.py
命令行下运行:
python demo.py
# 创建策略
#coding=utf-8
from xtquant.xttrader import XtQuantTrader, XtQuantTraderCallback
from xtquant.xtquant import StockAccount
from xtquant import xtconstant
class MyXtQuantTraderCallback(XtQuantTraderCallback):
def on_disconnected(self):
"""
连接断开
:return:
"""
print("connection lost")
def on_stock_order(self, order):
"""
委托回报推送
:param order: XtOrder对象
:return:
"""
print("on order callback:")
print(order.stock_code, order.order_status, order.order_sysid)
def on_stock_asset(self, asset):
"""
资金变动推送
:param asset: XtAsset对象
:return:
"""
print("on asset callback")
print(asset.account_id, asset.cash, asset.total_asset)
def on_stock_trade(self, trade):
"""
成交变动推送
:param trade: XtTrade对象
:return:
"""
print("on trade callback")
print(trade.account_id, trade.stock_code, trade.order_id)
def on_stock_position(self, position):
"""
持仓变动推送
:param position: XtPosition对象
:return:
"""
print("on position callback")
print(position.stock_code, position.volume)
def on_order_error(self, order_error):
"""
委托失败推送
:param order_error:XtOrderError 对象
:return:
"""
print("on order_error callback")
print(order_error.order_id, order_error.error_id, order_error.error_msg)
def on_cancel_error(self, cancel_error):
"""
撤单失败推送
:param cancel_error: XtCancelError 对象
:return:
"""
print("on cancel_error callback")
print(cancel_error.order_id, cancel_error.error_id,
cancel_error.error_msg)
def on_order_stock_async_response(self, response):
"""
异步下单回报推送
:param response: XtOrderResponse 对象
:return:
"""
print("on_order_stock_async_response")
print(response.account_id, response.order_id, response.seq)
if __name__ == "__main__":
print("demo test")
# path为mini qmt客户端安装目录下userdata_mini路径
path = 'D:\\迅投极速交易终端 睿智融科版\\userdata_mini'
# session_id为会话编号,策略使用方对于不同的Python策略需要使用不同的会话编号
session_id = 123456
xt_trader = XtQuantTrader(path, session_id)
# 创建资金账号为1000000365的证券账号对象
acc = StockAccount('1000000365')
# 创建交易回调类对象,并声明接收回调
callback = MyXtQuantTraderCallback()
xt_trader.register_callback(callback)
# 启动交易线程
xt_trader.start()
# 建立交易连接,返回0表示连接成功
connect_result = xt_trader.connect()
print(connect_result)
# 对交易回调进行订阅,订阅后可以收到交易主推,返回0表示订阅成功
subscribe_result = xt_trader.subscribe(acc)
print(subscribe_result)
stock_code = '600000.SH'
# 使用指定价下单,接口返回订单编号,后续可以用于撤单操作以及查询委托状态
print("order using the fix price:")
fix_result_order_id = xt_trader.order_stock(acc, stock_code,
xtconstant.STOCK_BUY, 200, xtconstant.FIX_PRICE, 10.5, 'strategy_name',
'remark')
print(fix_result_order_id)
# 使用订单编号撤单
print("cancel order:")
cancel_order_result = xt_trader.cancel_order_stock(acc, fix_result_order_id)
print(cancel_order_result)
# 使用异步下单接口,接口返回下单请求序号seq,seq可以和on_order_stock_async_response
的委托反馈response对应起来
print("order using async api:")
async_seq = xt_trader.order_stock(acc, stock_code, xtconstant.STOCK_BUY,
200, xtconstant.FIX_PRICE, 10.5, 'strategy_name', 'remark')
print(async_seq)
# 查询证券资产
print("query asset:")
asset = xt_trader.query_stock_asset(acc)
if asset:
print("asset:")
print("cash {0}".format(asset.cash))
# 根据订单编号查询委托
print("query order:")
order = xt_trader.query_stock_order(acc, fix_result_order_id)
if order:
print("order:")
print("order {0}".format(order.order_id))
# 查询当日所有的委托
print("query orders:")
orders = xt_trader.query_stock_orders(acc)
print("orders:", len(orders))
if len(orders) != 0:
print("last order:")
print("{0} {1} {2}".format(orders[-1].stock_code,
orders[-1].order_volume, orders[-1].price))
# 查询当日所有的成交
print("query trade:")
trades = xt_trader.query_stock_trades(acc)
print("trades:", len(trades))
if len(trades) != 0:
print("last trade:")
print("{0} {1} {2}".format(trades[-1].stock_code,
trades[-1].traded_volume, trades[-1].traded_price))
# 查询当日所有的持仓
print("query positions:")
positions = xt_trader.query_stock_positions(acc)
print("positions:", len(positions))
if len(positions) != 0:
print("last position:")
print("{0} {1} {2}".format(positions[-1].account_id,
positions[-1].stock_code, positions[-1].volume))
# 根据股票代码查询对应持仓
print("query position:")
position = xt_trader.query_stock_position(acc, stock_code)
if position:
print("position:")
print("{0} {1} {2}".format(position.account_id, position.stock_code,
position.volume))
# 阻塞线程,接收交易推送
xt_trader.run_forever()
开通xtquant的方式可以咨询。
目前开户费率低,门槛低,提供技术支持与交流。
需要的朋友,可以扫码咨询:

备注开户 收起阅读 »
ptrade每天自动打新 (新股和可转债)附python代码
ptrade软件-量化-回测 里,新建策略,复制全文粘贴进去,周期选分钟,再到交易里新增交易。可以参见文末图片。
交易页面
程序运行返回代码:
点击查看大图
【如果没有打新额度或者没有开通对应的权限,会显示可收购为0】
底下的成功的是可转债申购成功。
然后可以到券商app上看看是否已经有自动申购成功的记录。 收起阅读 »
import time
def initialize(context):
g.flag = False
log.info("initialize g.flag=" + str(g.flag) )
def before_trading_start(context, data):
g.flag = False
log.info("before_trading_start g.flag=" + str(g.flag) )
def handle_data(context, data):
if not g.flag and time.strftime("%H:%M:%S", time.localtime()) > '09:35:00':
# 自动打新
log.info("自动打新")
ipo_stocks_order()
g.flag = True
def on_order_response(context, order_list):
# 该函数会在委托回报返回时响应
log.info(order_list)
交易页面
程序运行返回代码:
点击查看大图
【如果没有打新额度或者没有开通对应的权限,会显示可收购为0】
底下的成功的是可转债申购成功。
然后可以到券商app上看看是否已经有自动申购成功的记录。 收起阅读 »
ptrade回测和实盘支持可转债日内tick/分时吗?
是支持的。
比如下面的部分代码片段,是用于导出可转债的日内分时数据的。最低颗粒,按照一分钟k线获取。 ptrade里面能够获取到的最小频率单位。
然后在handle_data里面加入相关的order就可以交易了。(上面的代码主要是保存tick/分时数据到mysql,实际并没有进行交易。) 收起阅读 »
比如下面的部分代码片段,是用于导出可转债的日内分时数据的。最低颗粒,按照一分钟k线获取。 ptrade里面能够获取到的最小频率单位。
def initialize(context):
# 初始化策略
g.code_mapper = date_code_mapper()
log.info(g.code_mapper)
g.error_set=set()
def last_n_day(date,n,origin_fmt,target_fmt):
return (datetime.datetime.strptime(date, origin_fmt) - datetime.timedelta(days=n)).strftime(target_fmt)
def post_fix(code):
return code + '.SZ' if code.startswith('12') else code + '.SS'
def handle_data(context, data):
date=context.blotter.current_dt.strftime('%Y%m%d')
if date not in g.code_mapper:
return
security_list=g.code_mapper.get(date)
for code in security_list:
code=post_fix(code)
try:
df = get_history(1, frequency='1m', field=['open' ,'high','low','close'], security_list=code, fq='pre',include=False)
except Exception as e:
if code not in g.error_set:
log.info(e)
log.info('代码获取数据 出错{}'.format(code))
g.error_set.add(code)
continue
df['ticker']=code
g.result.append(df)
def after_trading_end(context, data):
#engine = DBS
db = DBSelector()
conn = db.get_engine()
date=context.blotter.current_dt.strftime('%Y%m%d%H%M')
if len(g.result)==0:
return
df = pd.concat(g.result)
try:
df.to_sql('minute_info',con=conn,if_exists='append')
except Exception as e:
log.info(e)
g.result=
def before_trading_start(context, data):
g.result=
然后在handle_data里面加入相关的order就可以交易了。(上面的代码主要是保存tick/分时数据到mysql,实际并没有进行交易。) 收起阅读 »
ciso8601 性能对比 datetime 默认库
In [1]: import datetime, aniso8601, iso8601, isodate, dateutil.parser, arrow, ciso8601
In [2]: ds = u'2014-01-09T21:48:00.921000'
In [3]: %timeit ciso8601.parse_datetime(ds)
1000000 loops, best of 3: 204 ns per loop
In [4]: %timeit datetime.datetime.strptime(ds, "%Y-%m-%dT%H:%M:%S.%f")
100000 loops, best of 3: 15 µs per loop
In [5]: %timeit dateutil.parser.parse(ds)
10000 loops, best of 3: 122 µs per loop
In [6]: %timeit aniso8601.parse_datetime(ds)
10000 loops, best of 3: 28.9 µs per loop
In [7]: %timeit iso8601.parse_date(ds)
10000 loops, best of 3: 42 µs per loop
In [8]: %timeit isodate.parse_datetime(ds)
10000 loops, best of 3: 69.4 µs per loop
In [9]: %timeit arrow.get(ds).datetime
10000 loops, best of 3: 87 µs per loop
In [1]: import datetime, aniso8601, iso8601, isodate, dateutil.parser, arrow, ciso8601
In [2]: ds = u'2014-01-09T21:48:00.921000+05:30'
In [3]: %timeit ciso8601.parse_datetime(ds)
1000000 loops, best of 3: 525 ns per loop
In [4]: %timeit dateutil.parser.parse(ds)
10000 loops, best of 3: 162 µs per loop
In [5]: %timeit aniso8601.parse_datetime(ds)
10000 loops, best of 3: 36.8 µs per loop
In [6]: %timeit iso8601.parse_date(ds)
10000 loops, best of 3: 53.5 µs per loop
In [7]: %timeit isodate.parse_datetime(ds)
10000 loops, best of 3: 82.6 µs per loop
In [8]: %timeit arrow.get(ds).datetime
10000 loops, best of 3: 104 µs per loop
Even with time zone information, ciso8601 is 70x as fast as aniso8601.
Tested on Python 2.7.10 on macOS 10.12.6 using the following modules:
ciso8601 是纳秒级别的,如果要对上千万的数据操作,建议使用ciso这个C库。
收起阅读 »
ubuntu 安装ciso8601库 失败, 已解决
ubuntu20; ciso8601库是一个高性能的时间解析库,基于C底层写的。pip install ciso8601 报错 :
看样子,是setuptool的问题。 为了避免动这个默认库,可以安装降级版本的ciso。
pip install ciso8601==1.0.7
收起阅读 »
Collecting setuptools>=40.8.0
WARNING: Retrying (Retry(total=4, connect=None, read=None, redirect=None, status=None)) after connection broken by 'ReadTimeoutError("HTTPSConnectionPool(host='files.pythonhosted.org', port=443): Read timed out. (read timeout=15)")': /packages/a4/53/bfc6409447ca024558b8b19d055de94c813c3e32c0296c48a0873a161cf5/setuptools-63.2.0-py3-none-any.whl
Downloading setuptools-63.2.0-py3-none-any.whl (1.2 MB)
━━━━╸ 0.1/1.2 MB 102.5 kB/s eta 0:00:11
ERROR: Exception:
Traceback (most recent call last):
File "/tmp/pip-standalone-pip-oas0cddc/__env_pip__.zip/pip/_vendor/urllib3/response.py", line 435, in _error_catcher
yield
File "/tmp/pip-standalone-pip-oas0cddc/__env_pip__.zip/pip/_vendor/urllib3/response.py", line 516, in read
data = self._fp.read(amt) if not fp_closed else b""
File "/tmp/pip-standalone-pip-oas0cddc/__env_pip__.zip/pip/_vendor/cachecontrol/filewrapper.py", line 90, in read
data = self.__fp.read(amt)
File "/home/xda/miniconda3/envs/cpy/lib/python3.9/http/client.py", line 462, in read
n = self.readinto(b)
File "/home/xda/miniconda3/envs/cpy/lib/python3.9/http/client.py", line 506, in readinto
n = self.fp.readinto(b)
File "/home/xda/miniconda3/envs/cpy/lib/python3.9/socket.py", line 704, in readinto
return self._sock.recv_into(b)
File "/home/xda/miniconda3/envs/cpy/lib/python3.9/ssl.py", line 1241, in recv_into
return self.read(nbytes, buffer)
File "/home/xda/miniconda3/envs/cpy/lib/python3.9/ssl.py", line 1099, in read
return self._sslobj.read(len, buffer)
socket.timeout: The read operation timed out
During handling of the above exception, another exception occurred:
Traceback (most recent call last):
File "/tmp/pip-standalone-pip-oas0cddc/__env_pip__.zip/pip/_internal/cli/base_command.py", line 167, in exc_logging_wrapper
status = run_func(*args)
File "/tmp/pip-standalone-pip-oas0cddc/__env_pip__.zip/pip/_internal/cli/req_command.py", line 205, in wrapper
return func(self, options, args)
File "/tmp/pip-standalone-pip-oas0cddc/__env_pip__.zip/pip/_internal/commands/install.py", line 341, in run
requirement_set = resolver.resolve(
File "/tmp/pip-standalone-pip-oas0cddc/__env_pip__.zip/pip/_internal/resolution/resolvelib/resolver.py", line 94, in resolve
result = self._result = resolver.resolve(
File "/tmp/pip-standalone-pip-oas0cddc/__env_pip__.zip/pip/_vendor/resolvelib/resolvers.py", line 481, in resolve
state = resolution.resolve(requirements, max_rounds=max_rounds)
File "/tmp/pip-standalone-pip-oas0cddc/__env_pip__.zip/pip/_vendor/resolvelib/resolvers.py", line 348, in resolve
self._add_to_criteria(self.state.criteria, r, parent=None)
File "/tmp/pip-standalone-pip-oas0cddc/__env_pip__.zip/pip/_vendor/resolvelib/resolvers.py", line 172, in _add_to_criteria
if not criterion.candidates:
File "/tmp/pip-standalone-pip-oas0cddc/__env_pip__.zip/pip/_vendor/resolvelib/structs.py", line 151, in __bool__
return bool(self._sequence)
File "/tmp/pip-standalone-pip-oas0cddc/__env_pip__.zip/pip/_internal/resolution/resolvelib/found_candidates.py", line 155, in __bool__
return any(self)
File "/tmp/pip-standalone-pip-oas0cddc/__env_pip__.zip/pip/_internal/resolution/resolvelib/found_candidates.py", line 143, in <genexpr>
return (c for c in iterator if id(c) not in self._incompatible_ids)
File "/tmp/pip-standalone-pip-oas0cddc/__env_pip__.zip/pip/_internal/resolution/resolvelib/found_candidates.py", line 47, in _iter_built
candidate = func()
File "/tmp/pip-standalone-pip-oas0cddc/__env_pip__.zip/pip/_internal/resolution/resolvelib/factory.py", line 215, in _make_candidate_from_link
self._link_candidate_cache[link] = LinkCandidate(
File "/tmp/pip-standalone-pip-oas0cddc/__env_pip__.zip/pip/_internal/resolution/resolvelib/candidates.py", line 291, in __init__
super().__init__(
File "/tmp/pip-standalone-pip-oas0cddc/__env_pip__.zip/pip/_internal/resolution/resolvelib/candidates.py", line 161, in __init__
self.dist = self._prepare()
File "/tmp/pip-standalone-pip-oas0cddc/__env_pip__.zip/pip/_internal/resolution/resolvelib/candidates.py", line 230, in _prepare
dist = self._prepare_distribution()
File "/tmp/pip-standalone-pip-oas0cddc/__env_pip__.zip/pip/_internal/resolution/resolvelib/candidates.py", line 302, in _prepare_distribution
return preparer.prepare_linked_requirement(self._ireq, parallel_builds=True)
File "/tmp/pip-standalone-pip-oas0cddc/__env_pip__.zip/pip/_internal/operations/prepare.py", line 428, in prepare_linked_requirement
return self._prepare_linked_requirement(req, parallel_builds)
File "/tmp/pip-standalone-pip-oas0cddc/__env_pip__.zip/pip/_internal/operations/prepare.py", line 473, in _prepare_linked_requirement
local_file = unpack_url(
File "/tmp/pip-standalone-pip-oas0cddc/__env_pip__.zip/pip/_internal/operations/prepare.py", line 155, in unpack_url
file = get_http_url(
File "/tmp/pip-standalone-pip-oas0cddc/__env_pip__.zip/pip/_internal/operations/prepare.py", line 96, in get_http_url
from_path, content_type = download(link, temp_dir.path)
File "/tmp/pip-standalone-pip-oas0cddc/__env_pip__.zip/pip/_internal/network/download.py", line 146, in __call__
for chunk in chunks:
File "/tmp/pip-standalone-pip-oas0cddc/__env_pip__.zip/pip/_internal/cli/progress_bars.py", line 53, in _rich_progress_bar
for chunk in iterable:
File "/tmp/pip-standalone-pip-oas0cddc/__env_pip__.zip/pip/_internal/network/utils.py", line 63, in response_chunks
for chunk in response.raw.stream(
File "/tmp/pip-standalone-pip-oas0cddc/__env_pip__.zip/pip/_vendor/urllib3/response.py", line 573, in stream
data = self.read(amt=amt, decode_content=decode_content)
File "/tmp/pip-standalone-pip-oas0cddc/__env_pip__.zip/pip/_vendor/urllib3/response.py", line 538, in read
raise IncompleteRead(self._fp_bytes_read, self.length_remaining)
File "/home/xda/miniconda3/envs/cpy/lib/python3.9/contextlib.py", line 137, in __exit__
self.gen.throw(typ, value, traceback)
File "/tmp/pip-standalone-pip-oas0cddc/__env_pip__.zip/pip/_vendor/urllib3/response.py", line 440, in _error_catcher
raise ReadTimeoutError(self._pool, None, "Read timed out.")
pip._vendor.urllib3.exceptions.ReadTimeoutError: HTTPSConnectionPool(host='files.pythonhosted.org', port=443): Read timed out.
[end of output]
note: This error originates from a subprocess, and is likely not a problem with pip.
error: subprocess-exited-with-error
看样子,是setuptool的问题。 为了避免动这个默认库,可以安装降级版本的ciso。
pip install ciso8601==1.0.7
收起阅读 »
systemctl start influxdb 服务启动出错
又是一次典型的csdn踩坑记。。。。。
csdn的原文内容:
https://blog.csdn.net/xiangjai/article/details/123718413
安装上面的配置走,ubuntu的influxdb一直无法启动。
只好一个一个选项的排除:
最后发现那个bind-address的问题:
把端口哪行注释了,先用着。。。
csdn的原文内容:
https://blog.csdn.net/xiangjai/article/details/123718413
安装上面的配置走,ubuntu的influxdb一直无法启动。
只好一个一个选项的排除:
最后发现那个bind-address的问题:
把端口哪行注释了,先用着。。。
bolt-path = "/var/lib/influxdb/influxd.bolt"收起阅读 »
engine-path = "/var/lib/influxdb/engine"
enable = true
#bind-address = ":8086"
docker安装的podman报错
podman 报错
本来计划在docker里面安装一个docker,启动docker报错,
于是安装了一个podman,结果ps -a的时候报错。
唯一的办法,可能就是启动第一个docker的时候使用特权模式, privillage=True
收起阅读 »
ERRO[0000] 'overlay' is not supported over overlayfs
Error: error creating libpod runtime: 'overlay' is not supported over overlayfs: backing file system is unsupported for this graph driver
本来计划在docker里面安装一个docker,启动docker报错,
于是安装了一个podman,结果ps -a的时候报错。
唯一的办法,可能就是启动第一个docker的时候使用特权模式, privillage=True
收起阅读 »
映射端口 methodot 云主机 外部访问
默认情况下,methodot提供的免费云主机 固定了几个端口给外部访问:
如果我们做了web,要怎么映射出来呢?
很简单,只要把web端口改为8001 - 8005 之中的一个。
然后用上面表格中对应的端口映射来访问就可以了
比如下面的flask代码:
因为8001映射出去的端口是 33442,
所以你可以在浏览器访问你的主机:
curl http://xxxxxxxxxxxxx.methodot.com:33442/
话说,之前以为这个主机随时提桶跑路的,不过用到现在还好。
每一个应用都是一个docker镜像。 所以你的linux系统是无法使用 systemctl 控制服务启动的。
会包权限不够。 收起阅读 »
如果我们做了web,要怎么映射出来呢?
很简单,只要把web端口改为8001 - 8005 之中的一个。
然后用上面表格中对应的端口映射来访问就可以了
比如下面的flask代码:
from flask import Flask, jsonify
# 最基本的测试
app =Flask(__name__)
@app.route('/about')
def about():
return 'this is about page'
@app.route('/404')
def error_handle():
return '404 error'
@app.route('/')
def error_handle():
return jsonify({'code':100})
if __name__=='__main__':
app.run(host='0.0.0.0',port=8001,debug=True)
因为8001映射出去的端口是 33442,
所以你可以在浏览器访问你的主机:
curl http://xxxxxxxxxxxxx.methodot.com:33442/
话说,之前以为这个主机随时提桶跑路的,不过用到现在还好。
每一个应用都是一个docker镜像。 所以你的linux系统是无法使用 systemctl 控制服务启动的。
会包权限不够。 收起阅读 »
vmware player Unable to install all modules. See log for details
Vmware player
Unable to install all modules. See log for details
ubuntu下的vmware play经常会让更新模块。一起点击确认就可以正常编译更新。
而且一定要编译后才能打开虚拟机系统
但是奇怪的是,最近一次点击 更新,报错:
显示的英文错误信息:
Unable to install all modules. See log for details
看了日志:
报错信息在这里:
遇到问题后就google一番。
果然还是老外大神多。
翻了一个解决方案后,终于找到一个可行的。【所以必须的英语水平还是要的】
可行的方案:
去github下载最新的host-modules
https://github.com/mkubecek/vmware-host-modules
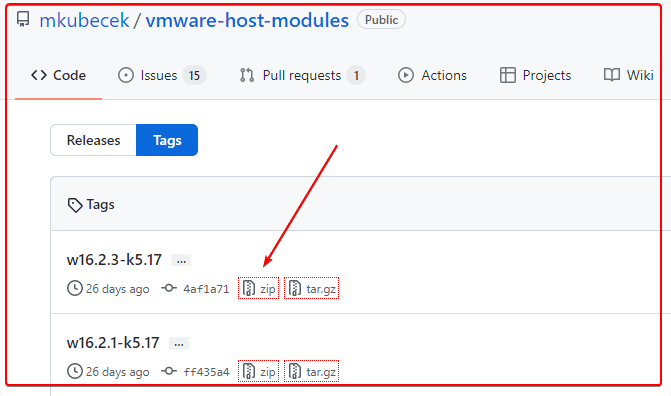
下载一个最新的。
然后解压:
得到以下文件
然后我们打包两个文件夹
这时,文件夹下多了2个tar的文件,vmmon.tar和vmnet.tar
然后拷贝到 目录:
/usr/lib/vmware.modules.source
之后可以直接编译:
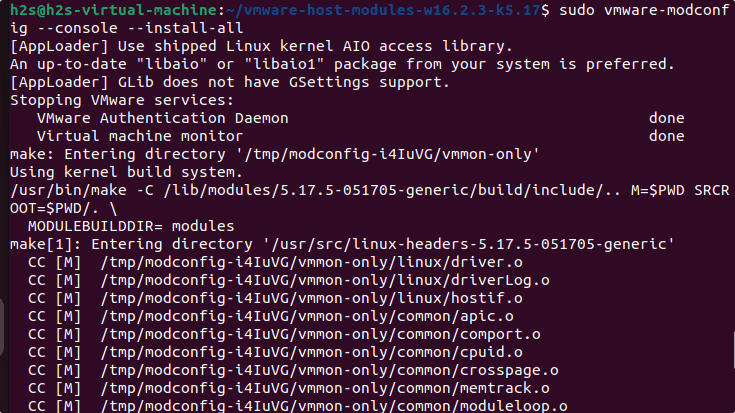
安装完成之后,再次打开vmware player就可以看到:
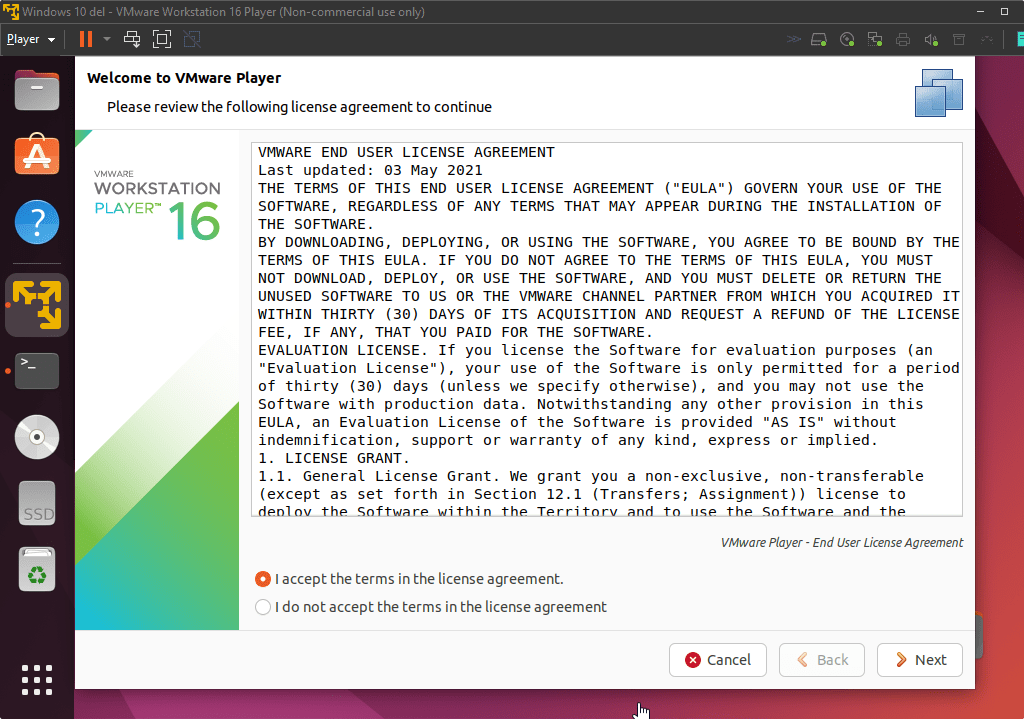
这样就是成功了。
收起阅读 »
Unable to install all modules. See log for details
ubuntu下的vmware play经常会让更新模块。一起点击确认就可以正常编译更新。
而且一定要编译后才能打开虚拟机系统
但是奇怪的是,最近一次点击 更新,报错:
显示的英文错误信息:
Unable to install all modules. See log for details
看了日志:
26 2022-07-15T02:15:09.595Z In(05) host-7426 /tmp/modconfig-PB4afO/vmmon-only/./include/vm_assert.h:372:22: note: in definition of macro ‘ASSERT_ON_COMPILE’
25 2022-07-15T02:15:09.595Z In(05) host-7426 372 | _Static_assert(e, #e); \
24 2022-07-15T02:15:09.595Z In(05) host-7426 | ^
23 2022-07-15T02:15:09.595Z In(05) host-7426 /tmp/modconfig-PB4afO/vmmon-only/./include/vm_asm_x86.h:215:7: note: in expansion of macro ‘ASSERT_ON_COMPILE_SELECTOR_SIZE’
22 2022-07-15T02:15:09.595Z In(05) host-7426 215 | ASSERT_ON_COMPILE_SELECTOR_SIZE(expr); \
21 2022-07-15T02:15:09.595Z In(05) host-7426 | ^~~~~~~~~~~~~~~~~~~~~~~~~~~~~~~
20 2022-07-15T02:15:09.595Z In(05) host-7426 /tmp/modconfig-PB4afO/vmmon-only/./include/vm_asm_x86.h:227:22: note: in expansion of macro ‘SET_SEGREG’
19 2022-07-15T02:15:09.595Z In(05) host-7426 227 | #define SET_GS(expr) SET_SEGREG(gs, expr)
18 2022-07-15T02:15:09.595Z In(05) host-7426 | ^~~~~~~~~~
17 2022-07-15T02:15:09.595Z In(05) host-7426 /tmp/modconfig-PB4afO/vmmon-only/common/task.c:2726:10: note: in expansion of macro ‘SET_GS’
16 2022-07-15T02:15:09.595Z In(05) host-7426 2726 | SET_GS(gs);
15 2022-07-15T02:15:09.595Z In(05) host-7426 | ^~~~~~
14 2022-07-15T02:15:09.595Z In(05) host-7426 make[2]: *** [scripts/Makefile.build:285: /tmp/modconfig-PB4afO/vmmon-only/common/task.o] Error 1
13 2022-07-15T02:15:09.595Z In(05) host-7426 make[2]: *** Waiting for unfinished jobs....
12 2022-07-15T02:15:09.595Z In(05) host-7426 make[1]: *** [Makefile:1875: /tmp/modconfig-PB4afO/vmmon-only] Error 2
11 2022-07-15T02:15:09.595Z In(05) host-7426 make: *** [Makefile:117: vmmon.ko] Error 2
10 2022-07-15T02:15:09.595Z In(05) host-7426 Using kernel build system.
9 2022-07-15T02:15:09.595Z In(05) host-7426 /tmp/modconfig-PB4afO/vmnet-only/driver.c: In function ‘VNetFileOpUnlockedIoctl’:
8 2022-07-15T02:15:09.595Z In(05) host-7426 /tmp/modconfig-PB4afO/vmnet-only/driver.c:966:7: warning: this statement may fall through [-Wimplicit-fallthrough=]
7 2022-07-15T02:15:09.595Z In(05) host-7426 966 | {
6 2022-07-15T02:15:09.595Z In(05) host-7426 | ^
5 2022-07-15T02:15:09.595Z In(05) host-7426 /tmp/modconfig-PB4afO/vmnet-only/driver.c:976:4: note: here
4 2022-07-15T02:15:09.595Z In(05) host-7426 976 | case SIOCGETAPIVERSION:
3 2022-07-15T02:15:09.595Z In(05) host-7426 | ^~~~
2 2022-07-15T02:15:09.595Z In(05) host-7426 Skipping BTF generation for /tmp/modconfig-PB4afO/vmnet-only/vmnet.ko due to unavailability of vmlinux
1 2022-07-15T02:15:09.595Z In(05) host-7426 Unable to install all modules. See log for details.
报错信息在这里:
host-7426 Skipping BTF generation for /tmp/modconfig-PB4afO/vmnet-only/vmnet.ko due to unavailability of vmlinux
host-7426 Unable to install all modules. See log for details
遇到问题后就google一番。
果然还是老外大神多。
翻了一个解决方案后,终于找到一个可行的。【所以必须的英语水平还是要的】
可行的方案:
去github下载最新的host-modules
https://github.com/mkubecek/vmware-host-modules
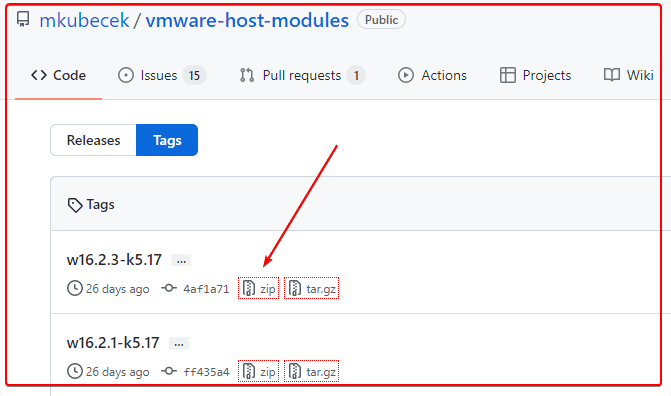
下载一个最新的。
然后解压:
unzip w16.2.3-k5.17.zip
得到以下文件
INSTALL
LICENSE
Makefile
README
vmmon-only
vmnet-only
然后我们打包两个文件夹
vmmon-only
vmnet-only
tar -cf vmmon.tar vmmon-only
tar -cf vmnet.tar vmnet-only
这时,文件夹下多了2个tar的文件,vmmon.tar和vmnet.tar
然后拷贝到 目录:
/usr/lib/vmware.modules.source
sudo cp -v vmmon.tar vmnet.tar /usr/lib/vmware/modules/source/
之后可以直接编译:
sudo vmware-modconfig --console --install-all
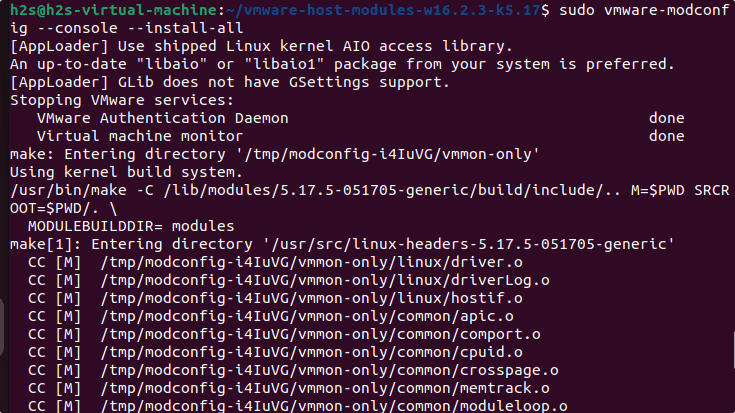
安装完成之后,再次打开vmware player就可以看到:
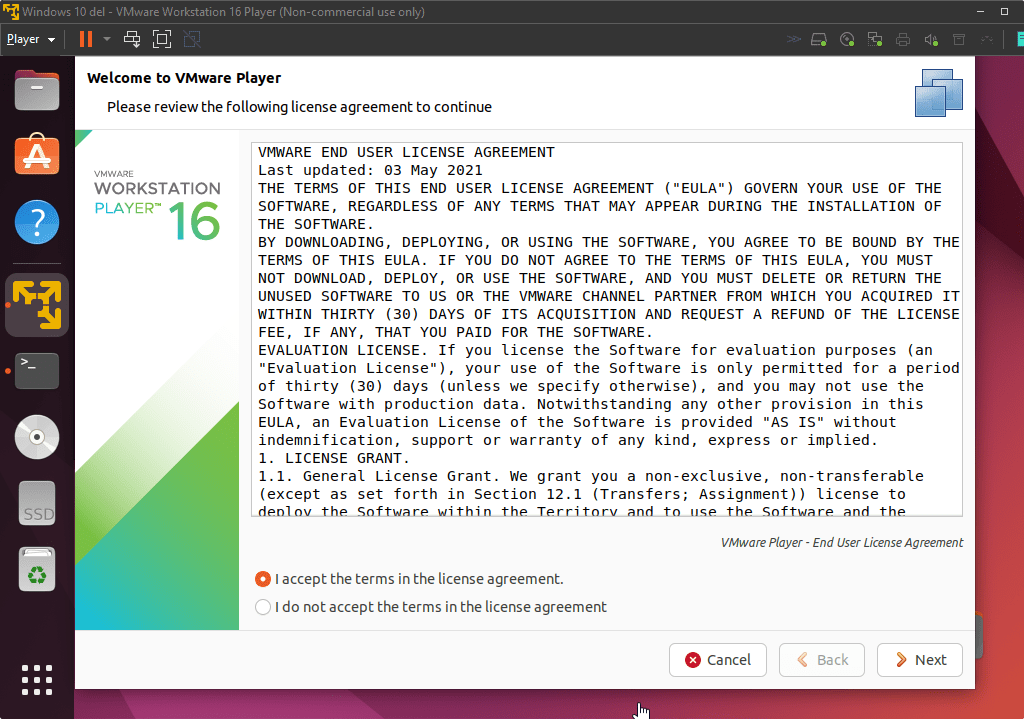
这样就是成功了。
收起阅读 »
linux监控shell进程是否运行,不运行的时候自动启动
脚本很简单。
保存为monitor.sh
给个执行权限
chmod +x monitor.sh
然后设置定时任务,
比如隔5分钟运行一次上面的脚本
*/5 * * * * monitor.sh
收起阅读 »
保存为monitor.sh
#!/bin/bash
line=$(ps -aux | grep -c /usr/sbin/ssh | grep -v "grep")
# 匹配的行数
if [ $line -eq 1 ];
then
sudo /etc/init.d/ssh restart
# 重启ssh服务
else
echo ssh is running....
# 向日志发送邮件,显示ssh运行中。。。
fi
给个执行权限
chmod +x monitor.sh
然后设置定时任务,
比如隔5分钟运行一次上面的脚本
*/5 * * * * monitor.sh
收起阅读 »
批量修改wordpress文章中的所有的链接
有时候你的文章中,某个地方的引用的链接失效了。需要你去替换一个新的链接。
假如只有2,3篇文章需要修改,那么就很简单,进入wordpress的后台进行修改。
但如果动辄几十篇,甚至上百上千篇文章,手动修改就工作量太大了,且容易出错。
那么可以选择后台数据库直接修改。
1. 先备份一下数据库,以防操作失误导致数据丢失。
2. 打开数据库软件,比如navicat
找到wp_post 这个表
运行下面的命令:
上面的sql命令就是把crop.png 的图片改为 resize.png 的图片的mysql命令。
修改万后,刷新一下缓存就可以了。 收起阅读 »
假如只有2,3篇文章需要修改,那么就很简单,进入wordpress的后台进行修改。
但如果动辄几十篇,甚至上百上千篇文章,手动修改就工作量太大了,且容易出错。
那么可以选择后台数据库直接修改。
1. 先备份一下数据库,以防操作失误导致数据丢失。
2. 打开数据库软件,比如navicat
找到wp_post 这个表
运行下面的命令:
UPDATE wp_posts set post_content = REPLACE(post_content,"crop.png","hxxy-resize.png") where post_content like '%crop.png%';
上面的sql命令就是把crop.png 的图片改为 resize.png 的图片的mysql命令。
修改万后,刷新一下缓存就可以了。 收起阅读 »
一创聚宽的实盘只支持每日开盘价和分钟交易
在一创的官网里面浏览到了一创上跑聚宽实盘的一些信息:
不支持虚拟机和xp系统,费率万2.5(这个实在要吐槽一下,太太太贵)
只支持股票和场内基金,不支持可转债。
只能跑一个策略。(额。。。无力吐槽)
执行周期最低的是分钟级别,没有秒级别。
其他量化平台实盘案例,可以参考本站其他文章。
收起阅读 »
1.交易佣金是多少?
A股万分之二点五,最低五元手续费;
货币类ETF买卖和申赎均无费用;
场内基金只能买卖不能申赎,交易费用根据客户交易佣金来定,有最低5元限制;
具体情况请联系一创咨询;
2.平台支持的交易频率是多少?
支持「每分」和「每天」两个交易频率
3.平台支持的交易品种有哪些?
目前支持A股、场内基金。
4.可以同时跑几个实盘策略?
目前每个用户可以运行1个实盘策略,需要更多权限请联系自己的客户经理申请。
5.其他问题如何咨询?
查看常见问题及实盘说明文档
点击聚宽官网的在线客服免费咨询
6.请问实盘支持哪些系统
请您使用win7、8、9、10系统和mac系统进行实盘账户的绑定操作。目前暂时不支持win xp等系统和虚拟机。
不支持虚拟机和xp系统,费率万2.5(这个实在要吐槽一下,太太太贵)
只支持股票和场内基金,不支持可转债。
只能跑一个策略。(额。。。无力吐槽)
执行周期最低的是分钟级别,没有秒级别。
其他量化平台实盘案例,可以参考本站其他文章。
收起阅读 »
ptrade的run_interval定时执行或者handle_data周期运行
在这几个函数里面打上标识,输出线程名字。
可以知道,他们是通过多线程触发的。 每一次运行的线程名字都不一样。
所以在里面操作一些共享变量的时候,最好加锁操作。
比如:
可以知道,他们是通过多线程触发的。 每一次运行的线程名字都不一样。
所以在里面操作一些共享变量的时候,最好加锁操作。
比如:
def query_offset(self,start,count):收起阅读 »
sqlite_str = 'select code,open,current from {} limit {},{}'.format(self.table_name,start,count)
cursor = self.db.cursor()
with lock:
try:
cursor.execute(sqlite_str)
except Exception as e:
log.info(e)
self.db.rollback()
else:
return cursor.fetchall()
aria2c 不能下载https的文件
下载命令:
aria2c https:// openresty.org/download/openresty-1.21.4.1-win64.zip
只要把https改为http就可以了。(前提是完整没有把http跳转到https)
aria2 http: //openresty.org/download/openresty-1.21.4.1-win64.zip
不过这个办法不是长久之计,要解决这个问题,需要你重新编译ariac2, 编译的时候添加 ssl参数就可以啦
进入ariac2的源码目录:
收起阅读 »
aria2c https:// openresty.org/download/openresty-1.21.4.1-win64.zip
$ aria2c
https: //openresty.org/download/openresty-1.21.4.1-win64.zip
07/10 10:58:58 [NOTICE] Downloading 1 item(s)
07/10 10:58:58 [ERROR] CUID#7 - Download aborted. URI=https: //openresty.org/download/openresty-1.21.4.1-win64.zip
Exception: [AbstractCommand.cc:351] errorCode=1 URI=https: //openresty.org/download/openresty-1.21.4.1-win64.zip
-> [InitiateConnectionCommandFactory.cc:87] errorCode=1 https is not supported yet.
07/10 10:58:58 [NOTICE] Download GID#b9bc95619990e7e4 not complete:
Download Results:
gid |stat|avg speed |path/URI
======+====+===========+=======================================================
b9bc95|ERR | n/a|
https: //openresty.org/download/openresty-1.21.4.1-win64.zip
http: //openresty.org/download/openresty-1.21.4.1-win64.zip
Status Legend:
(ERR):error occurred.
aria2 will resume download if the transfer is restarted.
If there are any errors, then see the log file. See '-l' option in help/man page for details.
只要把https改为http就可以了。(前提是完整没有把http跳转到https)
aria2 http: //openresty.org/download/openresty-1.21.4.1-win64.zip
不过这个办法不是长久之计,要解决这个问题,需要你重新编译ariac2, 编译的时候添加 ssl参数就可以啦
进入ariac2的源码目录:
./configure --with-openssl接着:
make && sudo make install然后就可以啦
收起阅读 »
python sqlite3 多线程 批量写入 【代码】
1. 随机生成一个数组数据
2. 在多线程里面批量插入数据
几个关注点:
sqlite3.connect(_type, check_same_thread=False) 要设置为False
批量写的时候,记得要加锁
假如不加锁会出错:
收起阅读 »
2. 在多线程里面批量插入数据
几个关注点:
sqlite3.connect(_type, check_same_thread=False) 要设置为False
批量写的时候,记得要加锁
import datetime
import random
import sqlite3
import threading
import logging as log
import time
lock = threading.Lock()
class SQLiteDBCls:
def __init__(self, cache=True):
_type = ":memory:"
self.db = sqlite3.connect(_type, check_same_thread=False)
self.table_name = 'tick_data'
def create_index(self):
cmd = 'CREATE INDEX code_ix ON {} (current)'.format(self.table_name)
with lock:
try:
cursor = self.db.cursor()
cursor.execute(cmd)
except Exception as e:
log.info(e)
self.db.rollback()
else:
self.db.commit()
def create_table(self):
# cursor = self.db.cursor()
cmd = 'create table if not exists {} (id INTEGER PRIMARY KEY AUTOINCREMENT,code text,open double,current time)'.format(
self.table_name)
with lock:
try:
cursor = self.db.cursor()
cursor.execute(cmd)
except Exception as e:
log.info(e)
self.db.rollback()
else:
self.db.commit()
def add(self, code, price, t):
cmd = 'insert into {} (code,open,current) values (?,?,?);'.format(self.table_name)
with lock:
try:
cursor = self.db.cursor()
cursor.execute(cmd, (code, price, t))
except Exception as e:
log.info(e)
self.db.rollback()
else:
self.db.commit()
def batch_add(self, data):
# 批量加入
print('===========',threading.current_thread().getName())
# log.info(threading.current_thread().getName())
cmd = 'insert into {} (code,open,current) values (?,?,?)'.format(self.table_name)
with lock:
try:
cursor = self.db.cursor()
cursor.executemany(cmd, data)
except Exception as e:
log.info(e)
self.db.rollback()
else:
self.db.commit()
def result(self):
cmd = 'select count(*) from `{}`'.format(self.table_name)
with lock:
try:
cursor = self.db.cursor()
cursor.execute(cmd)
except Exception as e:
log.info(e)
self.db.rollback()
else:
return cursor.fetchone()
def data_gen():
minute = 6000
code = ['123011.SS','110010.SS','112111.SS']
for i in range(minute):
current = (datetime.datetime.now()+datetime.timedelta(minutes=i)).strftime('%H:%M:%D')
data_list =
for c in code:
price = 5+random.random()+120
data = (c,price,current)
data_list.append(data)
yield data_list
# time.sleep(0.5)
app = SQLiteDBCls(cache=True)
app.create_table()
app.create_index()
def data_validation():
print(app.result())
app.sync_up()
def multithread_mode():
total_count = 0
thread_list =
for d in data_gen():
print(d)
total_count+=len(d)
# app.batch_add(d)
t=threading.Thread(target=app.batch_add,args=(d,))
thread_list.append(t)
for t in thread_list:
t.start()
for t in thread_list:
t.join()
print(total_count)
if __name__=='__main__':
multithread_mode()
data_validation()
假如不加锁会出错:
File "/home/xda/miniconda3/envs/cpy/lib/python3.9/threading.py", line 910, in run
self._target(*self._args, **self._kwargs)
File "/home/xda/github/stock_strategy/sqlite_issue_debug.py", line 77, in batch_add
self.db.commit()
Exception in thread Thread-3824:
Exception in thread Thread-3826:
Traceback (most recent call last):
File "/home/xda/miniconda3/envs/cpy/lib/python3.9/threading.py", line 973, in _bootstrap_inner
sqlite3.OperationalError: cannot commit - no transaction is activeTraceback (most recent call last):
File "/home/xda/github/stock_strategy/sqlite_issue_debug.py", line 72, in batch_add
cursor.executemany(cmd, data)
sqlite3.InterfaceError: Error binding parameter 0 - probably unsupported type.
收起阅读 »
低门槛开通量化交易接口Ptrade QMT (入金1万即可,AA级大券商)
(如果按证监会对证券公司的分类结果,(98家券商中,AA级只有15家,没有AAA级),算是头部券商,具备一定的实力)
该券商为AA级券商,GJ证券,腾讯入股的A股券商
该券商营业部最近对量化交易软件推广期,对开通门槛做了大幅降低, 目前只需要入金1W,既可以开通量化交易接口与软件,有Ptrade和QMT两个。 二者支持python语言编写策略,支持tick,分钟线,日线,等级别的实盘交易。
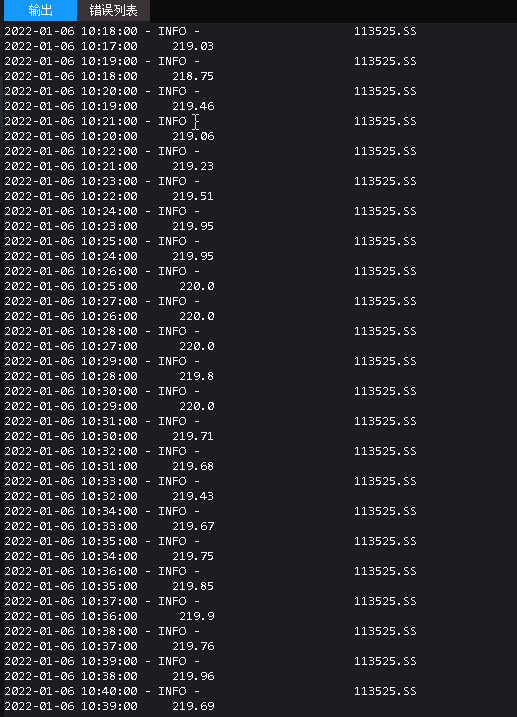
点击查看大图
Ptrade为云端部署,QMT为本地部署(你的策略只会放着本地,使用本地python运行)
点击查看大图
该QMT支持虚拟机内运行,也就是可以在云主机(腾讯云,阿里云这些服务器上)运行。
QMT
点击查看大图
目前开通门槛低,时间有限,需要的朋友抓紧时间开通,过了这个时间就没有下次机会了。
交易费率: 量化没有流量费。(针对该营业部开户用户)
股票万一
可转债(新规) 沪:十万分之四点四,深:十万分之四
基金:万0.5
没有流量费
需要的朋友可以加微信咨询开通:备注 一万量化开户 (否则不通过或者没有低门槛介绍)
收起阅读 »
该券商为AA级券商,GJ证券,腾讯入股的A股券商
该券商营业部最近对量化交易软件推广期,对开通门槛做了大幅降低, 目前只需要入金1W,既可以开通量化交易接口与软件,有Ptrade和QMT两个。 二者支持python语言编写策略,支持tick,分钟线,日线,等级别的实盘交易。
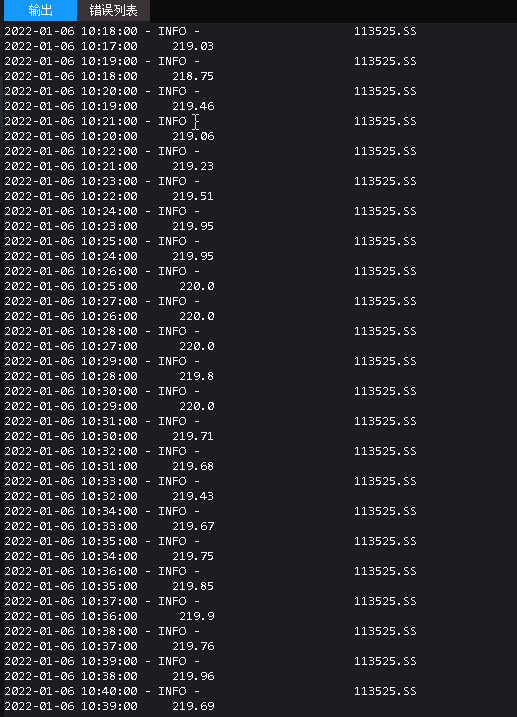
点击查看大图
Ptrade为云端部署,QMT为本地部署(你的策略只会放着本地,使用本地python运行)
点击查看大图
该QMT支持虚拟机内运行,也就是可以在云主机(腾讯云,阿里云这些服务器上)运行。
QMT
点击查看大图
目前开通门槛低,时间有限,需要的朋友抓紧时间开通,过了这个时间就没有下次机会了。
交易费率: 量化没有流量费。(针对该营业部开户用户)
股票万一
可转债(新规) 沪:十万分之四点四,深:十万分之四
基金:万0.5
没有流量费
需要的朋友可以加微信咨询开通:备注 一万量化开户 (否则不通过或者没有低门槛介绍)

qmt如何获取高频数据(大于3秒间隔的行情)
对于可转债日内交易的朋友,喜欢追涨杀跌。
可转债天生T+0, 适合使用量化工具进行操作,速度快,下单稳,快。
对于qmt而言,可以设置定时器,run_time.
你可以设置1毫秒执行一次,笔者也试过,贼快。 然后你就打算在间隔1毫秒里面获取行情,你就可以获取近乎实时的行情数据了吗? 注意,拿实时行情数据使用
想多了。
即使你设置1毫秒,而行情数据更新是3s更新一次,你在3s的间隔内,无论你用多高的频率获取,拿到的数据还是一个快照,3秒的快照。
也就是一般的操作,是无法突破快于3秒的行情。
那么如何突破呢。
使用订阅行情配合L2数据即可。
不过不一定所有的券商都支持L2数据。而且需要费用开通。
实际外面很多tushare,akshare数据源,他们的时间更新间隔也是大于3s的。 而集思录的数据源更新速度更慢,之前测试过,大约6s。
那么使用订阅行情如何获取快于3s的数据呢?
待续,持续更新ing。。。。
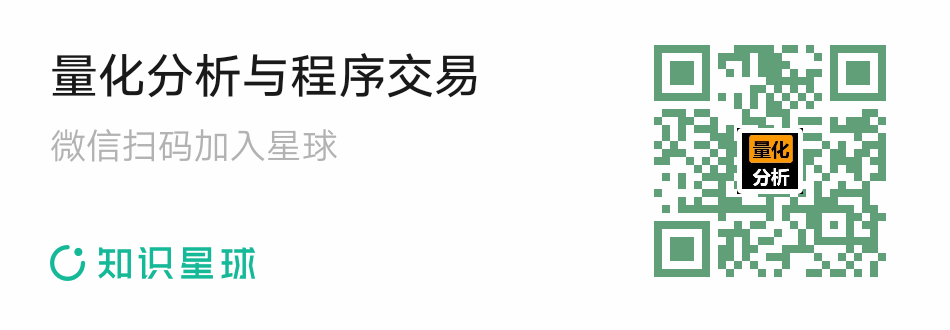
收起阅读 »
可转债天生T+0, 适合使用量化工具进行操作,速度快,下单稳,快。
对于qmt而言,可以设置定时器,run_time.
你可以设置1毫秒执行一次,笔者也试过,贼快。 然后你就打算在间隔1毫秒里面获取行情,你就可以获取近乎实时的行情数据了吗? 注意,拿实时行情数据使用
获取分笔数据 ContextInfo.get_full_tick()这个函数。
想多了。
即使你设置1毫秒,而行情数据更新是3s更新一次,你在3s的间隔内,无论你用多高的频率获取,拿到的数据还是一个快照,3秒的快照。
也就是一般的操作,是无法突破快于3秒的行情。
那么如何突破呢。
使用订阅行情配合L2数据即可。
不过不一定所有的券商都支持L2数据。而且需要费用开通。
实际外面很多tushare,akshare数据源,他们的时间更新间隔也是大于3s的。 而集思录的数据源更新速度更慢,之前测试过,大约6s。
那么使用订阅行情如何获取快于3s的数据呢?
待续,持续更新ing。。。。
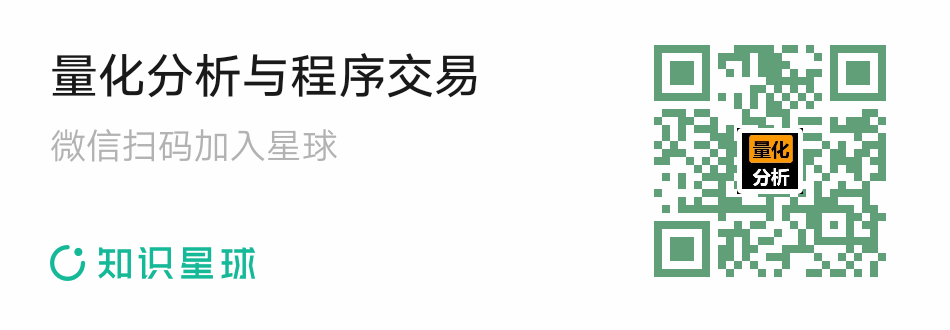
收起阅读 »